A research team of philosophers and computer scientists at the University of Cambridge is examining the ability of language models like GPT-3 to detect informal fallacies.
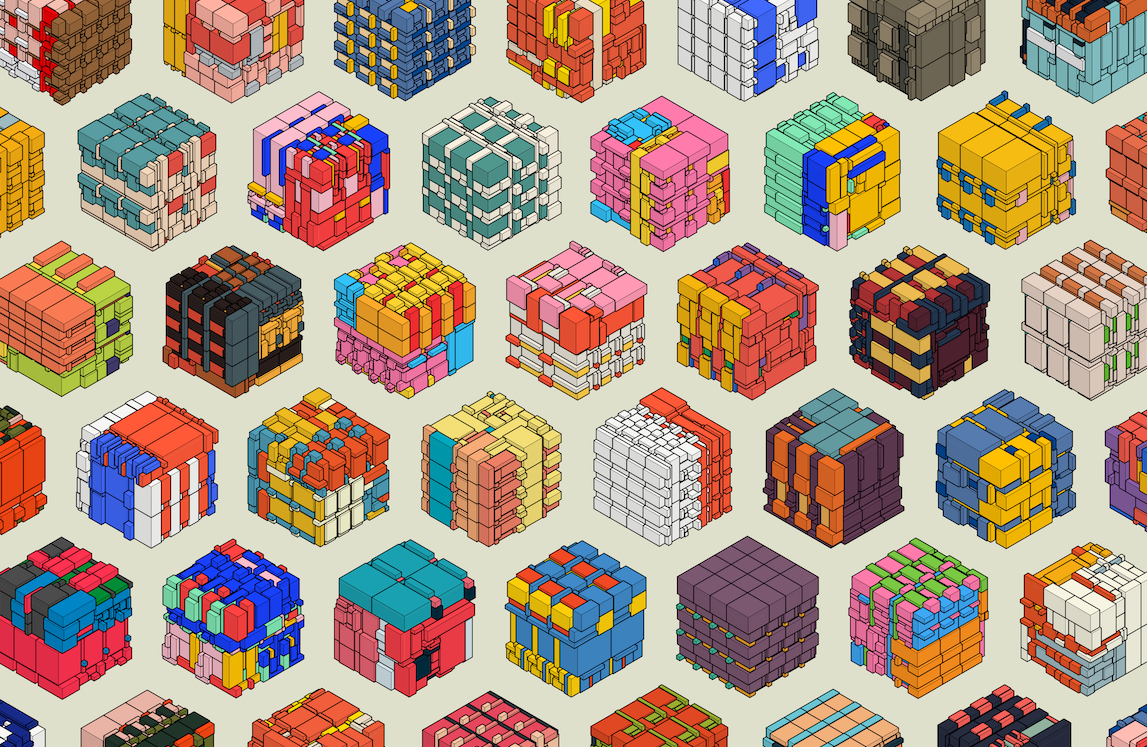
Why we need your help: To avoid subjectively biased datasets and obtain a larger set of samples, we’re reaching out to philosophers and those with related skills to obtain more examples of good and bad cases of informal reasoning, with the bad cases ideally exemplifying one or another of the most common informal fallacies typically taught in undergraduate reasoning courses. We kindly request that these be original examples not to be found anywhere online (in case they’ve already been part of the training data for the relevant models!). It shouldn’t take more than 5-10 minutes of your time, and you’re welcome to submit anywhere from 1 to 5 examples of fallacious and reasonable cases. Further details are available at the indicated link. All submitters who wish to make their names public will be acknowledged in materials submitted to BIG-bench.
Henry Shevlin, Research Associate at Cambridge’s Leverhulme Centre for the Future of Intelligence (CFI) has sent along details about the project and about how you can help:
What we’re doing: We’re a group of philosophers and computer scientists centered at the Leverhulme CFI at the University of Cambridge interested in developing benchmarks for AIs. Specifically, we’re working to submit a proposal to the new BIG-bench collaborative benchmark project (more information here).
An informal note on informal fallacies: Many philosophers (including some of us!) have previously been frustrated at the over-emphasis on teaching informal fallacies in many critical reasoning courses, which are sometimes prioritised to the detriment of other more valuable general reasoning skills such as identifying bias in sources. Moreover, we’re very sensitive to the fact that context (such as the speaker and audience) can matter a great deal for assessing the cogency of arguments, and we are certainly not trying to condense all informal reasoning into a box-checking exercise. Our hope instead is simply to start building one set of rudimentary tools that might eventually contribute to a broader set of benchmarks to help improve the reasoning abilities of LLMs. Your assistance is greatly appreciated!
Our specific hope at the moment is to assess the ability of these language models to detect informal fallacies—equivocations, ad hominem attacks, no-true-Scotsman arguments, faulty generalisations, and the like. While classifying fallacies sensibly and appropriately is far from trivial—many philosophy undergraduates struggle with it at the best of times—it has the advantage of being a relatively easy-to-operationalise aspect of informal reasoning that could help drive progress in AI natural language reasoning assessment in general. This latter project has the potential for significant social impact. If large scale language models could be developed that surpass human performance in categorising informal reasoning as good or bad, there may be many socially-impactful applications, ranging from more nuanced fact-checking to assistive tools to help students, policymakers, and journalists evaluate the cogency of their own arguments.
[Kjetil Golid, “Mint” (detail)]
Our specific hope at the moment is to assess the ability of these language models to detect informal fallacies—equivocations, ad hominem attacks, no-true-Scotsman arguments, faulty generalisations, and the like. While classifying fallacies sensibly and appropriately is far from trivial—many philosophy undergraduates struggle with it at the best of times—it has the advantage of being a relatively easy-to-operationalise aspect of informal reasoning that could help drive progress in AI natural language reasoning assessment in general. This latter project has the potential for significant social impact. If large scale language models could be developed that surpass human performance in categorising informal reasoning as good or bad, there may be many socially-impactful applications, ranging from more nuanced fact-checking to assistive tools to help students, policymakers, and journalists evaluate the cogency of their own arguments.
[Kjetil Golid, “Mint” (detail)]
Go here to submit a fallacy.
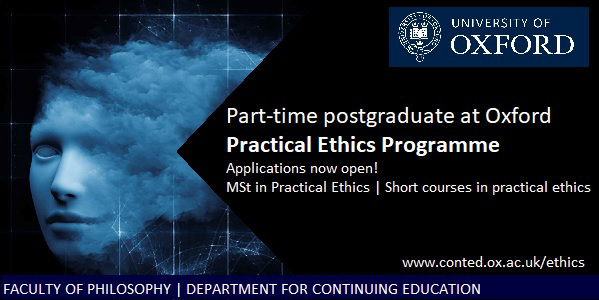