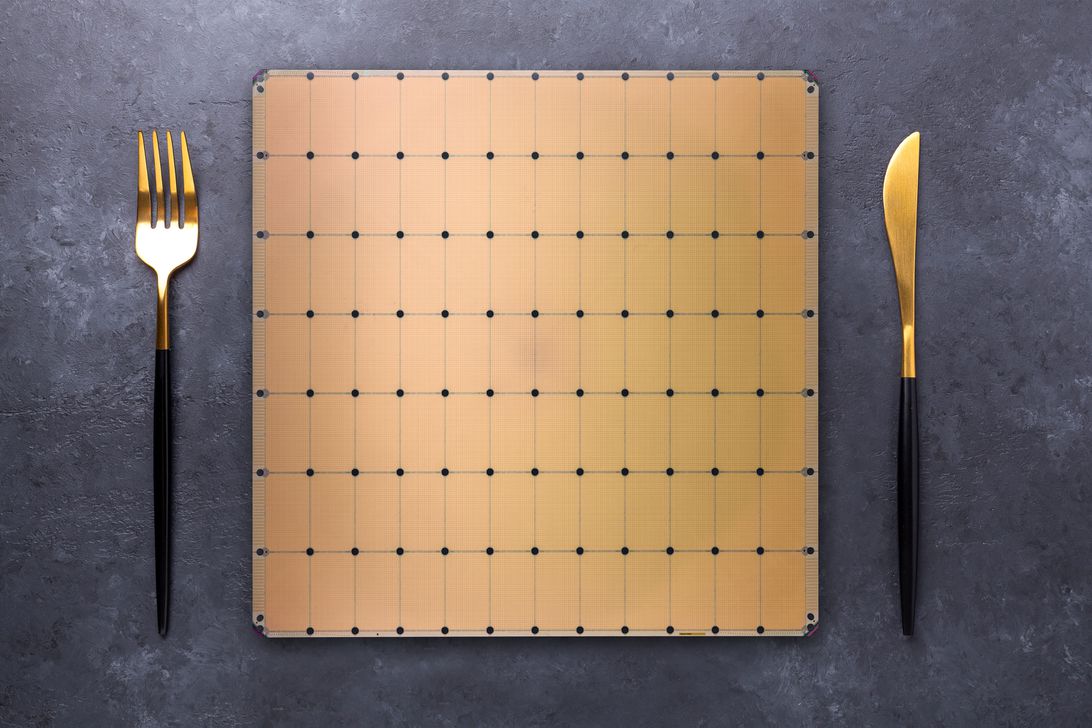
Cerebras
Cerebras is in still another league for chip size.
Just about every processor you’ve used, whether in your phone or watching a Netflix video, was originally among many cut from a circular wafer of silicon crystal 300mm across, or about 12 inches. The bigger the chip, the fewer fit on a wafer and more expensive it is to make, in part because there’s a higher likelihood one speck-size defect can ruin an entire unit. Typical general-purpose processors have billions of transistors. For example, the A14 processor in Apple’s iPhone 12 is 88 square millimeters and houses 11.8 billion transistors.
More powerful AI means advances that aren’t held back by the slow process of AI training. Open AI’s GPT-3, a high-profile AI system for generating human readable text, took four months to train. The second phase uses the model that’s been created, a task called inference, and applies it to new material. For example, the AI could be asked to recognize handwriting or spot malicious phishing emails. Computationally, inference is a much less difficult task though it still benefits from acceleration. Some of that happens on the chips Apple, Qualcomm, Intel and others design for consumer devices, and some in the sprawling “hyperscale” data centers that use thousands of servers for giant internet services like YouTube and Facebook.
Everyday computing jobs, like checking your email or streaming a video, mostly involve a single computing task, called a thread. Training an AI model, though, requires a system that can handle vastly more threads at a time.
Other AI chip companies also link their chips together — Tesla’s Dojo will group 3,000 D1 chips into a single unit it calls an Exapod. But Cerebras’ chip takes integration one step further.
Enormous AI chips
It’s a two-stage technology. Initially, a neural network learns to spot patterns in extremely large sets of carefully annotated data, such as labelled photos or speech recordings accompanied by accurate transcriptions. Training benefits from extremely powerful computing hardware.
More conventional AI chip work is underway at Intel, Google, Qualcomm and Apple, whose processors are designed to run AI software alongside lots of other tasks. But the biggest chips are coming from reigning power Nvidia and a new generation of startups, including Esperanto Technologies, Cerebras, GraphCore and SambaNova Systems. Their advancements could push AI far beyond its current role in screening spam, editing photos and powering facial recognition software.
Esperanto Technologies
AI chips, customized for the particular needs of AI algorithms and data, start from the same 300mm wafers as other processors but end up being just about the biggest in the business.
Cerebras counts pharmaceutical giant GlaxoSmithKline and supercomputing center Argonne National Laboratory as customers. The SWE-2 is the company’s second-generation processor.
Esperanto Technologies’ three-processor accelerator card plugs into a server’s PCIe expansion slot.
Even bigger: Cerebras AI chip
“AI workloads perform best with massive parallelism,” said 451 Group analyst James Sanders. That’s why AI acceleration processors are among the biggest in the business.
The result: a 2.6 trillion transistor processor covering a whopping 46,225 square millimeters — almost exactly the same area as an 11-inch iPad Pro, although squarer in shape. Interestingly, the company used AI software from chip design firm Synopsys to help optimize its own AI chip circuitry.
The chip startups unveiled their designs at the Hot Chips conference earlier this week. The conference took place just after Tesla detailed its ambitious Dojo AI training technology and the D1 processor at its heart. At that AI Day event, Tesla Chief Executive Elon Musk said the AI technology is good enough even to help a humanoid robot, the Tesla Bot, navigate the real world.
Esperanto’s ET-SOC-1 processor is 570 square millimeters with 24 billion transistors, co-founder Dave Ditzel said at Hot Chips. Tesla’s D1 processor, the foundation of the Dojo AI training system that it’s just now begun building, is 645 square millimeters with 50 billion transistors, said Dojo leader Ganesh Venkataramanan. Graphcore’s Colossus Mk2 at 823 square millimeters and 59 billion transistors.
Some of the new chips are enormous by the standards of the industry, which has prized miniaturization for decades. One chip for AI acceleration covers 46,225 square millimeters and is packed with 2.6 trillion transistors, the largest ever in the estimation of its designer, Cerebras.
AI tech: training and inference
Nvidia computer architect Ritika Borkar said in a Twitter thread that the flexibility of graphics chips helps the company deal with shorter cycles in AI, a field where techniques change roughly every 18 months.
Cerebras’ WSE-2 AI accelerator chip is made of a collection of rectangular processor elements, each as big as a very large conventional chip, that collectively occupy most of the area of an entire silicon wafer.
IBM
A host of established companies and fledgling startups are racing to build special-purpose chips to push the capabilities of artificial intelligence technology to a new level. The new chips are geared to help AI grasp the subtleties of human language and handle the nuances of piloting autonomous vehicles, like the ones carmaker Tesla is developing.
And the incumbent technology for training AI systems, high end graphics chips, remain powerful. Tesla’s current AI training system uses Nvidia’s graphics chips, for example.
AI chip challenges
Where ordinary processors are cut by the dozen or hundred from a 300mm wafer, Cerebras keeps the full wafer intact to make a single WSE-2 AI accelerator processor. Chipmaking equipment can only etch transistor circuitry on one relatively small rectangular area of the wafer at a time. But Cerebras designs each of those rectangular chip elements so they can communicate with each other across the wafer.
“The hyperscalers — places like Facebook and Amazon — really do benefit tremendously from AI,” which helps them more intelligently suggest posts you might want to see or products you might want to buy, said Insight 64 analyst Nathan Brookwood.
The Telum processor in IBM’s newest mainframes, arguably the most conservative server design in the industry, gets circuitry to boost AI software.